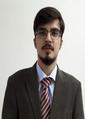
Maani Beigy
Tehran University of Medical Sciences, Iran
Title: Developing generalized resemblance models for precision/personalized psychiatry
Biography
Biography: Maani Beigy
Abstract
Background: Precision medicine emerges as the most important contemporary paradigm shift of medical practice but has several challenges in evidence synthesis and implementation for clinical practice. Because a modern physician, someone who practices precision medicine, should be able to quantify the possibility of having a particular condition precisely and to precisely choose the interventions for a particular individual.
Aim: We aimed to develop the required framework as a clinician-friendly, rapid, and low-cost clinical predictive method.
Methods: Generalized resemblance theory of evidence mainly rests upon generalized theory of uncertainty (GTU) which manages information as generalized constraints rather than limited statistical data, and also prototype resemblance theory of disease which defines the conditions (i.e., groups or subgroups) based on similarity measures with those prototypes and subgroups.
Results: We developed generalized resemblance linear models (GRLM) which are hybrid possibility-probability models that employ similarity measures to connect the high-dimensional variables with precisely defined outcomes. We used the data from NHANES study. The predictive power of GRLM was tested in detection of depression, in which GRLM (GRLM c-statistics=0.941, p-value<0.001; sensitivity=91.3%; specificity=94.9%) surpassed classical generalized linear models (c-statistics=0.797, p-value<0.001; sensitivity=78.3%; specificity=76.4%). The generalizability of data was demonstrated at the 2013-2014 cycle of NHANES.
Conclusion: GRLM seems to emerge as a feasible, robust and generalizable model for clinical prediction models in the realm of precision psychiatry.